“December is a wonderful time to be in Calcutta”, remarked the Director of ISI Kolkata, Prof. Sanghamitra Bandopadhyay, as she addressed the participants of a workshop on Machine Learning yesterday. In winter, Calcutta and the ISI campus seem to be embedded in a timescale of their own. The cool climate, with the leisure of end of semester break and the occasional chai in little earthen cups are an apt climax to a wonderful first leg of the PGDBA course here at ISI.
We had the opportunity to attend a lecture delivered by Dr. Sourav Sengupta held in the same Machine Learning workshop this week. Souravda, as he insisted we must call him when we first met in July, taught us a course titled ‘Computing for Data Science’ in this semester’s coursework. The topic of his workshop lecture on Tuesday was Linear Algebra and I couldn’t help but smile as I reminisced the most enjoyable classroom experience that I had in the past semester. When I decided on writing this piece I was lost for ideas on how to do justice, in the measure of just a few words, to a semester’s worth of constantly brilliant teaching. Just plain superlatives for Dr. Sourav Sengupta would not suffice to explain the privilege I feel in being his student.
There are many moments in class that I felt amazed as he walked us through a topic, drawing out concept after concept, most times exercising the last resource of our grey cells. With his characteristic smiles and pauses, he conducted the class like an orchestra maestro. He was always thoroughly prepared with the flow of his lectures that were packed with information and insight. Maybe trying to recap a few highlights would help reflect the memorable journey we’ve had in class.
Of our first few trysts in Souravda’s class was the one with Linear Algebra. He introduced matrix multiplication as an operation on vector spaces. Starting with linear combinations of vectors and the space they spanned, he guided us to a vector space representation of the linear least squares estimate of a system of equations. This might seem arcane to some, but the charm was in the ease with which he explained the ideas of multidimensional spaces and vectors in the context of something as simple as least squares approximation. Another of such crescendos was when he tied eigen values, Markov chains and the power method in a lecture on how Google first developed a base for ranking webpages called PageRank. It was almost a revelation when I first realised, “So this is a Markov chain!”
In another lecture, Souravda explained the notion of volume as it applies to higher dimensions. He compared a unit ball with a unit square. A unit square in two dimensions is completely contained inside a unit ball. The farthest that a square can get from the origin is root of 1/2 (1/4 +1/4 = 2/4) whereas a unit circle is at a fixed distance of 1. In three dimensions, a cuboid is still inside a sphere and the farthest it gets to is square root of 3/4. Now, as we move up to four dimensions, the farthest point inside the ‘box’ is at square root of 4/4 which is 1. So in four dimensions, the box just touches the ball but is still contained entirely inside it. “What happens, when we move higher up”, asked Souravda in class. It so happens that the unit box creeps out of the ball in the fifth dimension and further up, in still higher dimensions, most of the volume of the box is not contained inside the ball. This was just one aspect of the notion of distances that he discussed. In another class, we discussed the distance between two sentences or text corpuses to see how similar or different they were. And as measures of similarity of two sets, Souravda then introduced us to hash functions and MinHashing.
“How would you develop a system to choose one person from three people, uniformly at random, by using just the outcomes of coin tosses?” This is one of Prof. Bimal Roy’s favourite questions (previous Director and professor of MathStat department) that Souravda introduced in class with us. He then extended the discussion to choosing uniformly at random from n people and then on to storing streaming data in an efficient way.
PCA, SVD, Linear Regression and Regularisation, Clustering, Classification and Regression Trees, Bias-Variance Trade-off in learning algorithms, SVM, Recommender Systems, Expectation Maximisation are the other broad topics that he discussed at a constant level of commitment and brilliance.
And that is just his teaching. There’s another side to the person that is Souravda. His utmost sincerity and dedication, at times when it seemed unimaginable why a person should go so far out of his way to help, was an immeasurable gift during the course.
At times when he explained algorithms for certain applications, he would use the common refrain, “But can you do any better?” in trying to guide us to more efficient solutions. A lesson that Souravda’s students could take from his classes, of which his own dedication was sufficient evidence, was to keep asking themselves, “Can you do any better?”
Thank you and farewell, Dr. Sourav Sengupta.
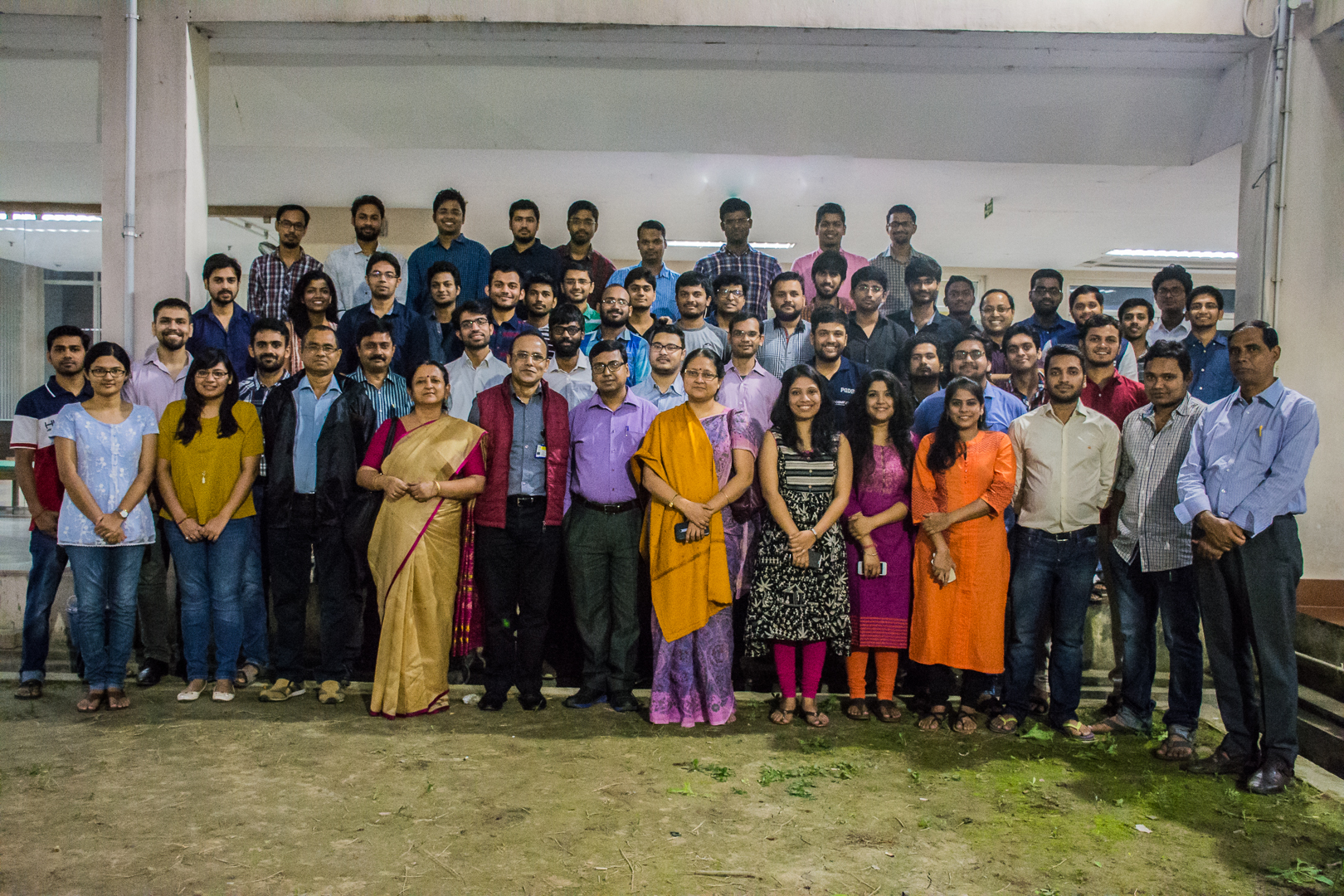
PGDBA batch of 2016-18 at Indian Statistical Institute, Kolkata
Be the first to comment